H2020-ICT-RIA-2019-“BeFerroSynaptic”-871737 “BEOL technology platform based on ferroelectric synaptic devices for advanced neuromorphic processors”
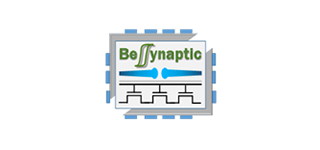
ACRONYM:
BeFerroSynaptic
LEADER:
Athanasios Dimoulas
START DATE:
01/01/2020
FUNDING SOURCE:
H2020