Machine Learning-aided Multiscale Modelling Framework for Polymer Membranes
https://cordis.europa.eu/project/id/101030668
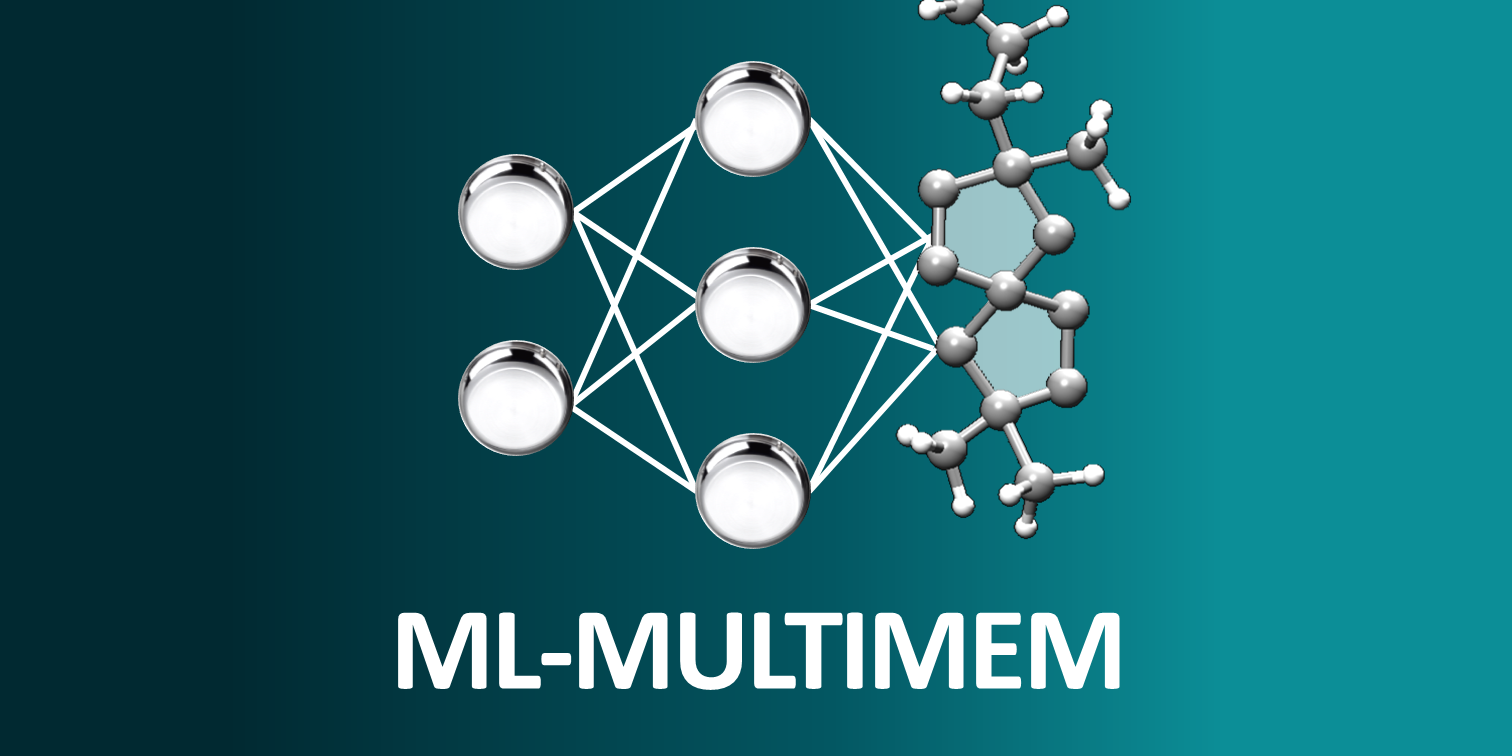
ACRONYM:
ML-MULTIMEM
START DATE:
15/11/2021
LATE DATE:
15/11/2023
FUNDING SOURCE:
H2020-MSCA-IF-2020
https://cordis.europa.eu/project/id/101030668
ACRONYM:
ML-MULTIMEM
START DATE:
15/11/2021
LATE DATE:
15/11/2023
FUNDING SOURCE:
H2020-MSCA-IF-2020
ML-MULTIMEM project targets the integration of artificial intelligence algorithms in multiscale molecular simulation methodologies for polymers. The goal of this project is to build a systematic modelling framework for advanced polymer materials, that are widely employed in numerous membrane separation applications, especially as gas separation media for carbon capture. Polymers are very challenging to simulate, due to the wide range of timescales that are present in these systems and require elaborate system-specific multiscale strategies. A hierarchical simulation strategy will be developed, encompassing atomistic, mesoscopic and continuum scales, integrating machine learning techniques. The artificial intelligence aided multi-scale approach proposed constitutes a generalized methodology for the efficient computational study of polymers. The synergy of unsupervised machine learning (ML) clustering techniques and neural networks (NN), will enable the extraction of accurate coarse-grained (CG) representations and force fields of the polymer systems, bringing this complex problem within computational reach. Optimized ML models will be integrated into Molecular Dynamics and innovative Monte Carlo simulations at the CG level, with the latter enabling the equilibration up to high molecular weight of polymers of complex chemical constitution, and the prediction of their micro- and macroscopic behaviour. Molecular simulation results will be integrated into macroscopic equation-of-state-based models, resulting in a bottom-up determination of the relevant process parameters for membrane separations (permeability and selectivity) in a wide range of conditions, for pure gases and gas mixtures. Systematic hierarchical modelling provides unique property prediction means, simultaneously shedding light on the mechanisms that are responsible for the materials end-use performance. This is a stepping stone towards the rational design of advanced processes from the molecular level all the way up to industrial applications, which in the present case involve novel separation technologies with great environmental impact.
The project is a collaboration between the Software and Knowledge Engineering Lab (SKEL) of the Institute of Informatics and Telecommunications (Dr Vangelis Karkaletsis and Dr George Giannakopoulos) and the Molecular Thermodynamics and Modelling of Materials Laboratory (MTMML) (Dr Niki Vergadou) of the Institute of Nanoscience and Nanotecnology of NCSR “Demokritos”. The project is funded under the Marie Skłodowska-Curie Actions programme (H2020-MSCA-IF-2020, ID:101030668) and Dr. Eleonora Ricci is the postdoctoral researcher awarded the Marie Skłodowska-Curie Postdoctoral Fellowship.
Related Work:
Nasikas, Dimitris, Ricci, Eleonora, Giannakopoulos, George, Karkaletsis, Vangelis, Theodorou, Doros N. and Vergadou, Niki, Investigation of Machine Learning-Based Coarse-Grained Mapping Schemes for Organic Molecules Article Number: 51, 2022 [doi] (PDF)
Ricci, Eleonora, Giannakopoulos, George, Karkaletsis, Vangelis, Theodorou, Doros N. and Vergadou, Niki, Developing Machine-Learned Potentials for Coarse-Grained Molecular Simulations: Challenges and Pitfalls Article Number: 51, 2022 [doi] (PDF)